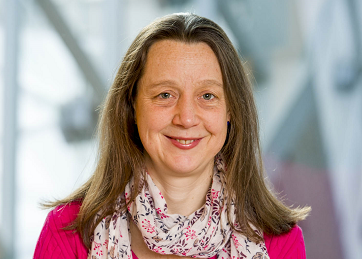
Carrie Rutland
Making the most of the UK Government’s incentives and grants for research and development should be an easy decision for every company or business. The incentives will either provide a welcome cash injection to your business or reduce your tax burden. With a two year deadline for a claim there is always a chance to submit or even review any projects or activities with a potential innovation or R&D aspect.
Any investment or project that results in improvements may qualify for some form of incentive. The outcome does not have to be a completely new product or process. Simply investing in a product or service to make it more efficient, cheaper or more sustainable may be enough to qualify for some R&D support. The activity may be central to your business model, or it could be an investment in your back back-office functions.
Any company in any sector and at any stage of its life cycle may be investing in projects, products or processes that qualify for R&D support.
The key to accessing these incentives and cash injections is getting the right advice. You need to understand the full range of R&D activities and projects that can qualify for government support. This requires a deep knowledge of the sources of support available and the respective criteria and application processes. It also requires a passion for and knowledge of the science and technology that is driving innovation in your business.
Our team of engineers, scientists, software developers and financial consultants will provide you with the knowledge and expertise to access all of the available incentives as effectively as possible. We will review all your recent activities as well as advise on all current and future projects.
You will benefit from our hard-earned understanding of what it takes to quickly and effectively submit successful claims with HMRC Our specialists have a deep knowledge of the legislation and guidance with practical experience of making claims in a wide range of sectors and negotiating these claims with HMRC. You will also see the advantages of our excellent and longstanding working relationship with HMRC.
Our approach to working with you is simple. We take the time to fully understand your business model and your operations. Our experience of working with businesses like yours is that this broad and comprehensive review allows us to identify all the opportunities for obtaining support and incentives in the most cost-effective manner. All too often, we find that businesses have missed out on cash payments or tax allowances because they have not identified all relevant costs in their applications.
Our clients also know that our fees for advising you on R&D will reflect the potential gains for you. It is always worthwhile talking to us about your potential R&D incentive.
The government encourages and incentivises innovation and R&D through a number of channels. The incentives take the form Research and Development tax credits and allowances such as patent box and other sector specific tax reliefs. Companies can also access a range of grants for innovation and growth projects.
We have provided more extensive information on the different incentives available for innovation and R&D on the accompanying pages.
You can also get in touch using the button below and we will get back to you to discuss your R&D and innovation needs and how we can help.